If you think that AI is the most significant disruption in human history, boy, do I have news for you.
It’s ain’t even close.
If you were born in Europe in the 1870s, your chances of survival to the age of 5 were basically 50-50, chances of you losing your mother at birth were ~20%, and chances of you seeing at least one famine in your life pretty much 100%. Chances of you seeing a plague in your lifetime were also ~100%, and chances of dying from it were pretty high too. And even if you didn’t fall to a plague, a small scratch could kill you through infection. Or a slightly unfresh fish or piece of meat you got on the market or bartered from a neighboring peasant.
And it was a norm. The misery of the human condition. The reason people went to church and prayed for a better afterlife and had 5-8 children was in hopes at least one of them would survive long enough to take care of them in their old age.
Fast forward ~60 years.
The Modern Biology
It’s 1930.
There are those pesky fascists and communists, but outside politics, life is pretty good.
Natural famines are pretty much a thing of the past. Even the Dust Bowl in the US was shrugged off like nothing. Thanks to the progress of agriculture, selective breeding of crops and livestock, mechanization of agriculture, and the discovery of fertilizers and chemical processes to synthesize them, food was always there, and if no one took it away from you, you had an abundance of it.
Penicillin was that new discovery that seemed to just kill bacteria and cure a bunch of diseases that were previously a death sentence – Syphilis to Boubonic Plague to Sepsis. However, thanks to the germ theory of diseases and the personal and public health measures it brought, you were unlikely to meet them in the first place. Rubber condoms protected you against Venerian Diseases. The general availability of soap – against typhoid, cholera, and a bunch of other diseases. Rubbing alcohol allowed you to disinfect your wounds and scratches, and the chances of you dying from them were pretty much zero. Refrigeration, ice boxes, and microscope-aided inspection made sure most of the food you were buying was safe.
You also had vaccines for the first diseases. Even if all else failed and you found yourself in the middle of smallpox, bubonic plague, cholera, or typhoid outbreaks, the power of modern medicine powered by science and vaccines protected you.
Vaccines for rabies, tetanus, pertussis, vitamins, and first intensive care made sure your kid would almost surely survive infancy and early childhood.
Basically, the paradise you were praying for as a child and a teenager – you have pretty much ascended to it as an adult.
And all of that was thanks to the synergy of chemical, agricultural, and medical revolutions, all driven by the core principles discovered by biology, notably microbiology, and genetics.
The Biology Revolution came. It was glorious, and its stewards – admired as modern gods
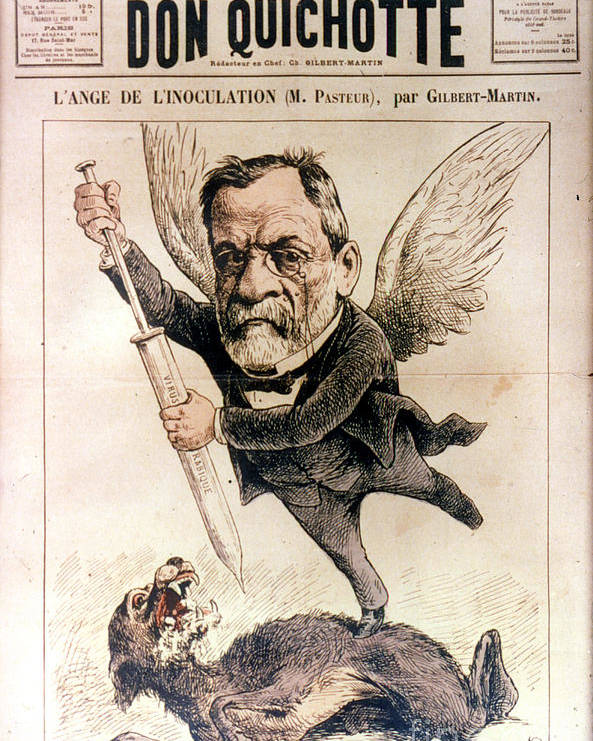
Eugenics
Unfortunately, limitless public admiration often attracts people who believe they are indeed better than everyone else and that they indeed deserve that admiration.
And for the Biology Revolution, those people were eugenists. Whereas the people who led the biological revolution were despised and prosecuted, eugenists were generally admired and revered.
They came at the apogee of the biology revolution, and to them, it had to go further and get rid the humankind of misery once and for all. And for that, they were going to use power and genetics to remove all the bad genes.
They believed that society had to get rid of ill, infirm, and disabled people to remove the genes that made people susceptible to those diseases.
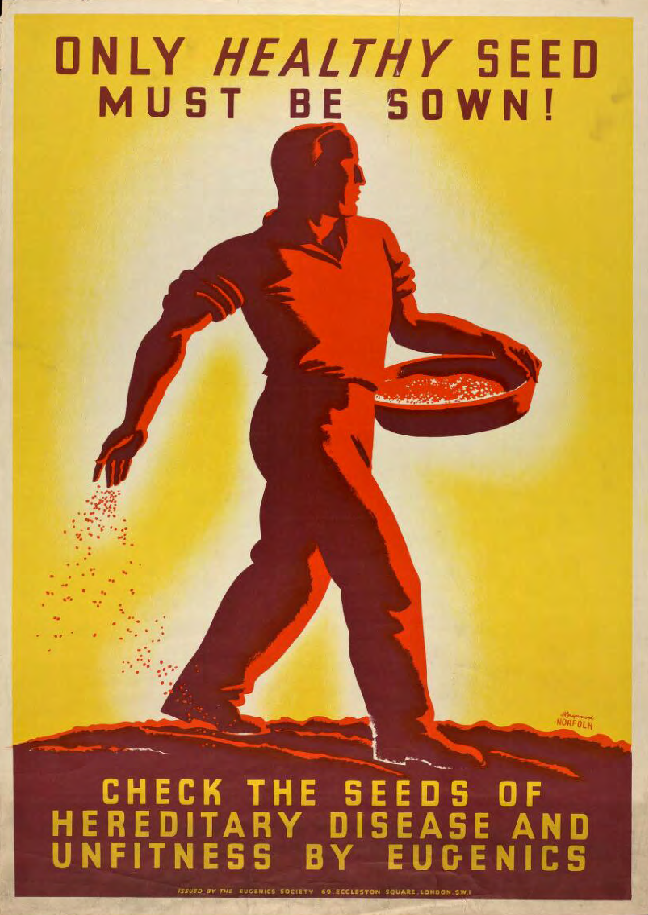
They believed that society had to get rid of deviant (read gay, lesbian, and trans) and criminal to remove the genes that made people deviant and criminal.
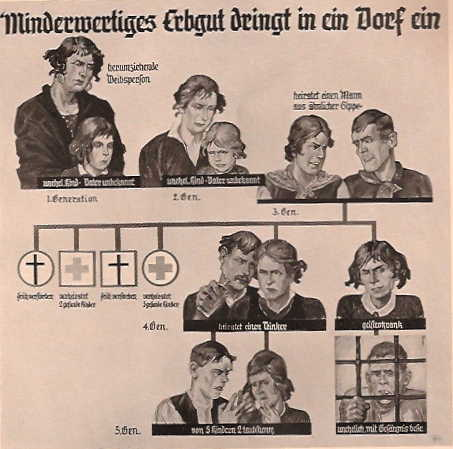
They believed that society had to get rid of the lazy, drunkards and not smart enough to remove the genes that made people lazy and stupid.
They believed the society had to get rid of the uncivilized (read Black, Aboriginal, Indian, Irish, Italians, Slavs, …), to remove genes that were making people uncivilized.
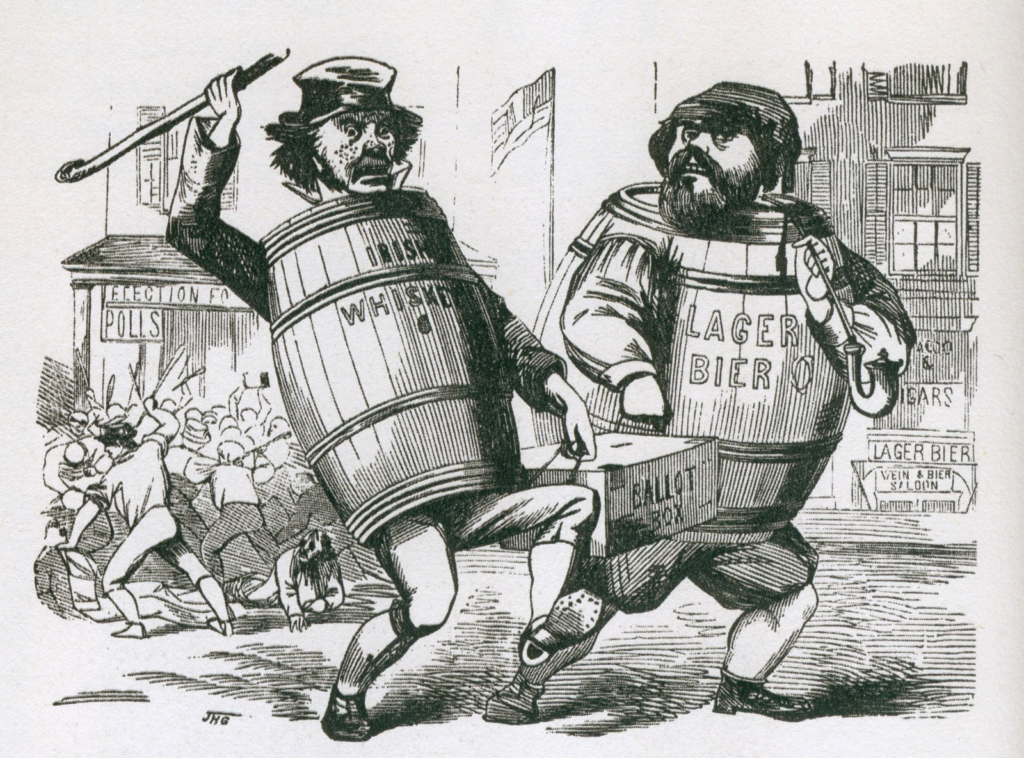
Basically, for them, society had to get rid of anyone who did not look like them – the motors of progress and the flag bearers of the Biology Revolution.
It sounds inhuman and borderline caricatural today. But we live in the post-WWII world, a world that witnessed the horrors of nazis and the horrors of actual attempts to put those ideas into action.
Back in the late 19th and early 20th century, pretty much every major scientific figure was a proponent of eugenics – from the “father of statistics and modern evolutionary synthesis” – Sir Ronald Fisher – to Sir Winston Churchill, “Teddy” Theodore Roosevelt, Graham “inventor of the telephone” Bell, WE Du Bois (yes, even though he was black himself) and John Maynard “Keynesian economics” Keynes.
They were the crème de la crème of the high society, generally seen as the most reputable and smartest people of their generation. They were ubermensch – captains of industry, politics, and science, able to anticipate the future, foresee what needed to be done, and act decisively to forge the destiny of humankind, standing above any old morality.
And yet, even if we set aside for a second the inhumanity of their views, they were plainly, simply, and unbelievably wrong in the most trivial way they should have themselves known.
The Original Sin of Eugenics
You probably have guessed that the sin in question is hubris.
Hubris of believing one was able to anticipate the future, and the hubris of conviction that this future somehow hinged on you.
Coming from a background in population genetics, my own academic genealogy traces directly to Sir Ronald Fisher. The guy who is one of the godfathers of eugenics and defended it and the nazis until early the 1960s – pretty much until the end of his life.
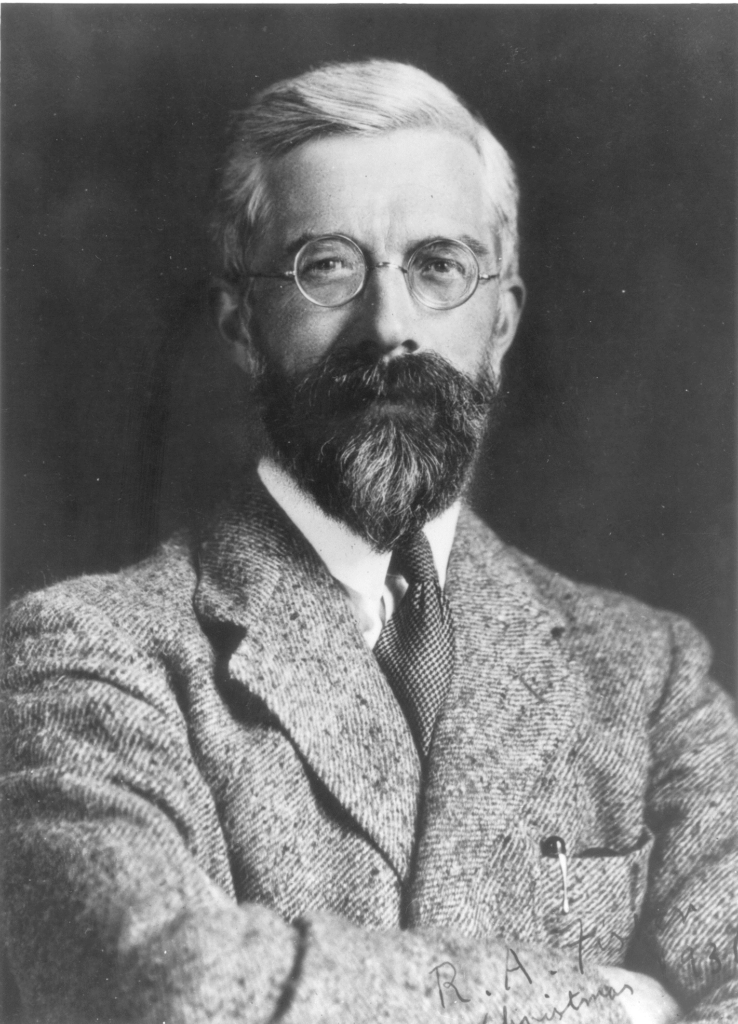
As a matter of fact, a big part of my Ph.D. thesis was building up on the tool he invented to perform the evolutionary theory Great Synthesis – Fisher’s Geometric Model of Evolution.
Because of that, I am extremely well familiar with the topic of eugenics and probably one of the best suited to talk about what exactly eugenicists got wrong – scientifically.
And it’s really stupid and trivial. As a matter of fact, Fisher himself proved it wrong in the 1920s with his Geometric Model – before he got on the eugenics train himself.
The thing is that from the point of view of evolution, there is no such thing as laziness or stupidity. Or deviance, criminality, civilization, or infirmity. There is just fitness.
And fitness is only defined with respect to the environment – the external conditions.
Full stop.
Those lazy no-goods? They are saving energy in the middle of the winter, given the lack of food and heating supplies.
That slightly autistic child doesn’t socialize with peers and doesn’t want to play team games? Boy, should you see them programming, prototyping, or building mechanical models.
That guy is totally ADHD and cannot for the life of his focus on anything of more than five seconds because he is distracted by anything moving? You should really see him mounting guard or trying to spot an animal to hunt in dense woods.
Those people have genetically transmittable sickle cell disease? Tough luck, but they are also going to survive malaria that is going to kill you.
That black skin means vitamin D deficiencies and troubles with kids developing in the northern latitudes? Tough luck, without it, you will die of sunburns and skin cancer close to the equator.
Etc…
Basically, eugenicists were a boy’s club that got too full of themselves and decided that the only environment that existed were the academic, political, and office halls, and since they were doing so well in them, surely anyone with any hope of achieving a high fitness would have to look like them.
Which sounds as unbelievably stupid as it is, but unfortunately, as is often the case, this stupidity escaped people whose self-esteem hinged on that stupidity. And as Max Plank put it – those people don’t change their point of view with time; their views will die with them. Science advances one funeral at a time.
And population genetics started advancing again only after Fisher’s one, in 1962. In late 1969, Kimura proposed a heretic idea that evolution might be aimless and not selecting for fitness (Neutral theory of evolution – which was actually critical to explain a number of empirical observations). In 1972 Gould and Eldridge put back the role of the environment and its change as a driver of evolution with their Punctuated Equilibrium theory of evolution. And finally, in the late 1980s and early 1990s, Gillespie and Orr introduced the Fitness Landscapes evolution model, which is the current theoretical evolution framework and a valuable tool that we use to study the evolution of multi-drug resistant pathogens and develop new drugs (including against COVID).
And in the current evolution theory, eugenics not only makes no sense, but it is also plain stupid. Imagining for a second that optimizing for a single environment has any value in the long run across a range of en environments is intuitively wrong. It’s not even clear how anyone could even come up with an idea, let alone people who studied evolution to start with.
And yet they did.
Worse than that. The vast majority of negative traits eugenicists were seeking to eliminate were the flip side of pretty big strengths.
Through eugenics, you eliminated sickle cell disease in northern latitudes? Surprise, surprise, global warming triggered by the industrial revolution has moved the Malaria way up north, and now you have millions of people dying, given no resistance genes remained. Oh, you did it in the 1950s, and you didn’t think it would matter because, certainly, we would do something to prevent global warming before it gets that bad?
You eliminated all deviance? Surprise, you also got rid of Alain Turing.
You eliminated all “uncivilized” genes? Congratulations, your population is now almost clonal, and a disease that manages to contaminate a single person can wipe out your entire population. Congrats, you should have learned a thing or two from banana plantations and the death of Gros Michel.
And you have absolutely no idea which flip sides will be critical in the future. Not only you have no idea now, no one can. In principle. No matter how smart, knowledgeable, or hard-thinking they are.
Because of impossibility theorems.
Somewhere around the same time as the population geneticists were deriving the general principles of evolutionary processes, logicians were trying to solve a recently discovered paradox in the magnificent and set theory that was promising to unify most, if not all, math – Russel’s paradox.
Unfortunately, rather than solving it, they proved that any logical system – assuming it was complex enough to contain basic arithmetic – was bound to have both contradictions that were impossible to predict and undecidable statements.
The statement above, in its more strict formulation, is known as Gödel’s incompleteness theorems and they were proven formally in the 1930s.
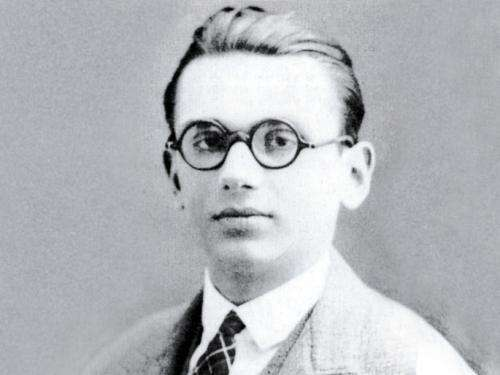
Basically, they mean that no matter how smart the eugenics ubermensch would be, how much they would already know, and no matter how much they would be able to reason, they won’t be able to anticipate the future.
Worse than that. Even if they tried to focus just on the most probable conditions rather than all of them, it won’t work out either – given that they can’t evaluate the probability of events they cannot decide in their logical system.
Once again, part of the impossibility theorems, the statement above in its more strict formulation is known as Solomonoff(-Kolmogorov) incompletness theorem and has been formally proven.
In other terms, no one can predict the future unless there are really good chances it would look like something you’ve seen before (aka sun rising up at the east in the morning).
Surprisingly, despite being proven in the 1930s, those results once again were ignored by eugenicists. Not because they didn’t know about them but because they didn’t care. Logicians were an annoying minority, whereas eugenicists were almost universally admired and ran the power circles. At least until the horrors of concentration camps – the culmination of the eugenicist logic – came to light at the end of WW2 and public opinion radically shifted.
Until then, if you were to go against eugenics in the early 20th century, you would have been pretty much doomed to be treated as paria by the intellectuals of your time.
If you would have argued it was inhuman, you would have been told that “it’s just math, and math cannot be wrong.”
If you had enough background knowledge to fight back on the axioms underlying the math used to justify eugenics, you would have been generally ignored because the reputation of eugenicists as flag-bearers of progress was unquestionable, and it would have only hurt your own reputation.
Science ended up moving forwards, and truth prevailed. But it wasn’t through the old guard changing their opinion. It was through them dying out.
As always, science advanced one funeral at a time. Funeral of someone that moved it forwards a mere 40-50 years prior.
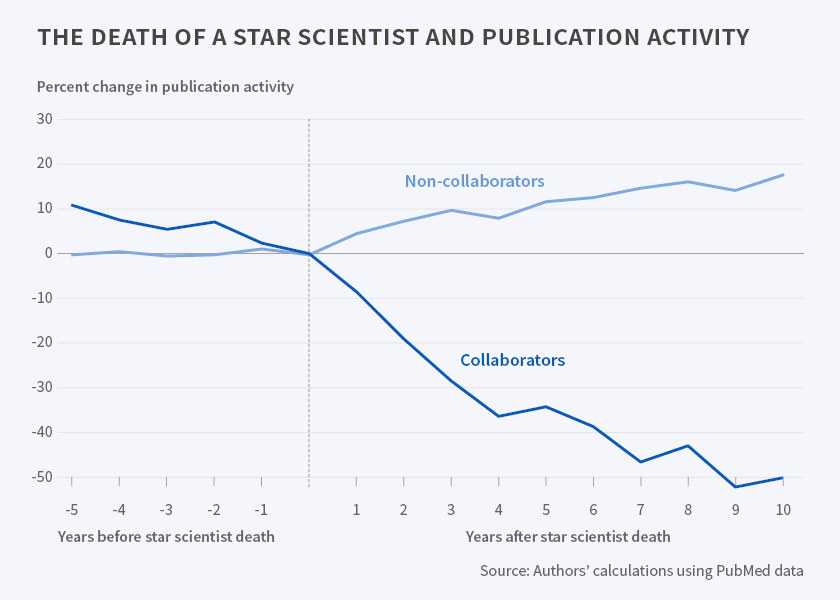
Longetrmist Effective Altruism
If you know anything about the modern Longermist Effective Altruism, the eugenics history must have felt eerily familiar.
I am not going to waste time by re-explaining who Longtermist EAs are – it has been extensively done by the mainstream media after several scandals involving its most visible figures (eg here, here, and here).
The biggest difference between Longtermist EA and eugenics is that the ubermensch is not human anymore. It’s AI. More specifically, AGI.
AGI will be able to foresee perfectly what needs to be done and act decisively to forge the destiny of humankind, standing above any old morality.
Their emergence and superiority to mere human mortals is once again “proven by math.”
If you think that AGI – or its seed – today’s AIs – are inhumane, well, “it’s just math, and math cannot be wrong.”
And once again, the Longtermist EAs are the captains of industry, politics, and academia, at least in appearance.
According to the Longtermist EA, the only thing that we can do is to invest all of our efforts to make sure that once AGI emerges, it will be “aligned,” so on its path to forging a new destiny above any morality, it spares the worthy humans and elevates them and their descendence into a pure bliss at the Known Universe level, with 10^23 human brains it can contain.
And them and only them, the Longtermist Effective Altruists, are smart and worthy enough to anticipate how AGI will develop and ensure it develops in the “right” direction. Or know what “right” means.
Basically, Longtermist EAs believe they are ubermensch by procurement.
Which, put that way, sounds stupid, and that’s because it actually is. For the very same reasons for which eugenics was stupid – we are basically building the AI BIG BROTHER optimized to assist and emulate the 80 000h; 80h/week self-realization work dudes and turn everyone else who isn’t one into one, assuming they have a high enough IQ; if not relegate them to “support roles.”
Except that somehow, they managed to sound even stupider than eugenicists, given all the talk of the galaxy-level 10^23 brains.
Provably, the degree of anticipation of the future its advocates are proscribing to AGI is provably impossible – due to the proved impossibility theorems.
Even if it was possible, the Longermist Effective Altruists provably will not be able to anticipate how it will develop and even less figure out what will be needed in the future – due to the impossibility theorems, once again.
What makes this even more frustrating is that Longtermist EA forums abound with computer science majors – who certainly have heard about at least one of the impossibility result – Turing’s halting problem, “No Free Lunch” theorem and extensively cite Solomonoff and Kolmogorov’s other work.
Something that, surprisingly, none of the 80+h/week crowd per week have not had the time to think about, certainly because they are too busy doing constant self-improvement to better improve AIs.
What is even more surprising is that none of them in those 80+h/week found the time to open a paleontology/geology book (or the corresponding Wikipedia pages) and think about whether current concepts of happiness, consciousness, or human brain could still hold at the timescales of galactic space travel.
FFS, 400 years the best world’s minds consensus on what the fittest human of the future would look like would have been “hardy, fit, endurant man able to survive on little food, resist diseases do a lot of physical work, not think too much or be too smart for his own good and be pious”. Pretty much all of those features are either neutral or deleterious for EA Longtermists these days.
Their today’s brain-on-a-stick view of a perfect human almost surely will look just as stupid 400 years from now.
A billion years into the future, when the humanity will reach the far corners of the known Universe? There is nothing we have today that could predict what life – let alone post-humans – would look like.
A billion years back, the earth was a frozen slush ball, with the most advanced life on earth being biofilm bacterial colonies. While some modern concepts that existed for life back then (inter-cellular communication, cell wall, RNA, organelles, …) are still relevant in organisms living today, there is nothing in what have been optimal growth conditions that transpose to higher organisms today.
With regards to organisms that will be around in a billion years, we are not much more complex than those biofilms. And even if we destroy ourselves into oblivion (through AI or not), the Universe will get back there in a couple of billion years more.
In the grand scheme of things, we do not matter; neither for universe or even carbo-based life.
The obsession of Longtermist EAs with timescale they are talking about makes no sense whatsoever – even if it is just with human survival. We can already nuke ourselves out of existence, and with global climate change, we are already driving a mass extinction event that we have a good chance of joining (remember that part about fitness being defined by the environment? Applies to humans as well). And otherwise, there are still good old errant asteroids or gamma-ray jets that can score a direct hit on the Earth and skip right to the final cutscene of the whole extinction process.
Basically, the AGI hype today has little more function than the claims of racial superiority a century ago – allowing a bunch of people to feel even more important and pull even more resources toward themselves.
And the tools they are using to achieve it – faux-formalism, pseudo-scientificism, and outright opponent suppression – are also the same.
“You feel like current AI is inhumane? Must feel bad to be so stupid you don’t understand that they are just math and math is never wrong”.
“Oh, the ML models are discriminative? They must be right, though – it’s a good decision to discriminate! Science and math tell us ML is right, so it must be right!”
I wish those were strawman arguments. Unfortunately, I heard them firsthand from Ronald Fishers of ML and AI (Yann, if you are reading, this is not a compliment).
It is not a coincidence either that AI and ML experts with enough background to cut through math BS get fired from their industry and research positions.
Unlike what you are led to believe, ethics teams that were silenced or fired – at Google, Microsoft, Facebook, … – are not woke hippy idealists that want to stop ML development. They are pretty good technical experts that are seeing the damage AI is already doing today and who also see AGI rhetoric for what it – a void multitude; a scarecrow to distract from real, current problems; the White Gorilla to stop you from thinking about Lakoff’s Pink Elephant of who trains the ML models, who calls them AI and what they are used for.
It is perhaps not a coincidence that Longtermist EAs always talk about the “alignment” of AIs but never mention to whose values they are aligned. It is perhaps not a coincidence that in their famous “Pause the Giant AI Experiments,” the Longtermist Future of Life Institute made a Freudian slip and added “loyal” as a condition to the AI research progress.
Without ever specifying the “Loyal to whom?” part.
Although the start of the list of signatories could give some idea on the subject, just as how well and by whom the Longtermist EA is funded, and who started their own AI development right after declaring that GPT is way too woke of an AI.
Eugenics is Directly Implied by the Longtermist Effective Altruism and is Implemented through Natalism
However, the eugenics is also connected to Longetermist Effective Altruism more directly.
Given that Longtermists EAs believe that they can anticipate the future and their focus on the well-being of future generations, one of the first “corrections” it makes sense to make is to remove congenital diseases or weaknesses. You know, the type that would make one unsuited or unwilling to work 80+h/week towards self-realization.
Yeah. Eugenics.
Not the concentration camps, of course – it’s too much of an untenable position politically (although less and less these days).
But definitely through “selecting the right seed” – which coincidentally happens to be one of the millionaires and billionaires who were “successful” and are donating to the Longtermist EA.
Perhaps it is not a coincidence that one of the most visible Longtermist EA figures are also natalists.
Such as our good Ol’ Musky with his ten children from three women. Children he is too busy to interact with while he is throwing billions out the nearest window.
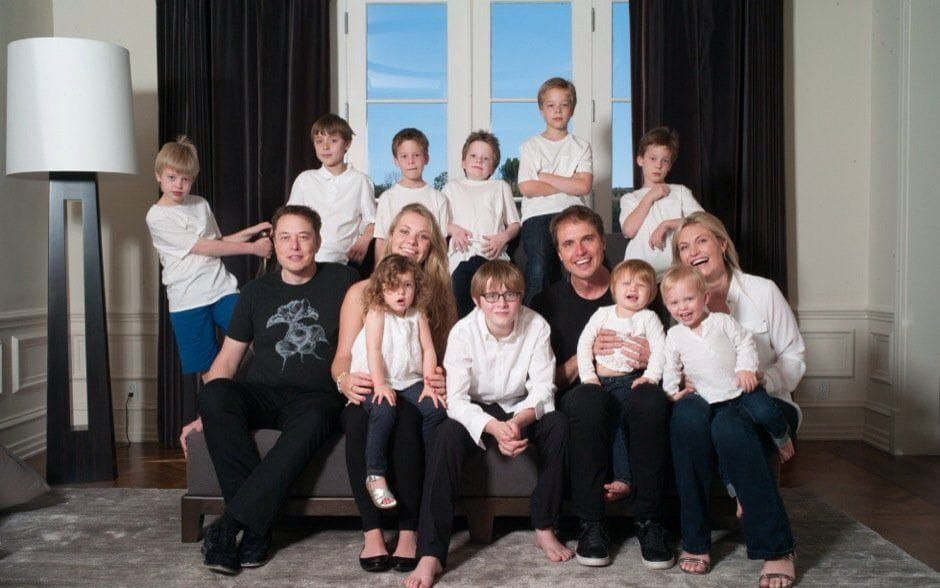
Or some other public figures from the tech community.
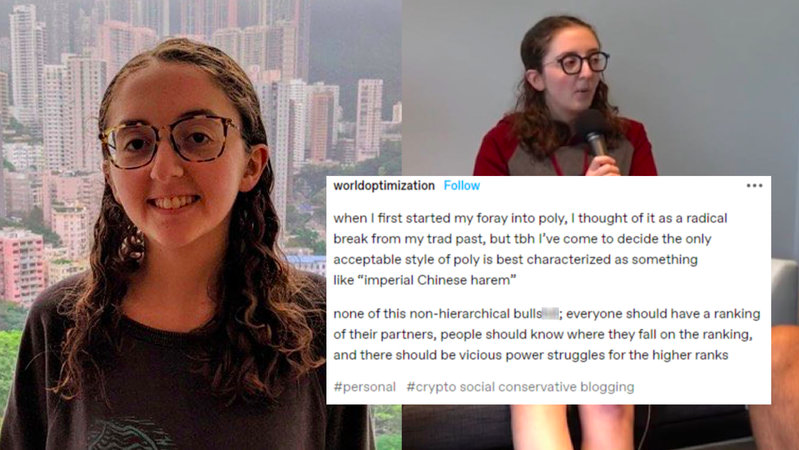
Funny how a lot of people decrying harems are all of a sudden fine with them when its billionaires doing it.
Instead of a conclusion.
Perhaps the most terrifying thing about eugenics is how widely it was supported by the people who were meant to be the smarts of their time.
And how hopelessly and obviously they were wrong.
And how impossible it was to go against them back at the time.
And how long it took after their death to put things straight again.
Oh, and the fact that some of them genuinely created entirely new fields of science almost entirely by themselves before hijacking that field and a good part of society with it.
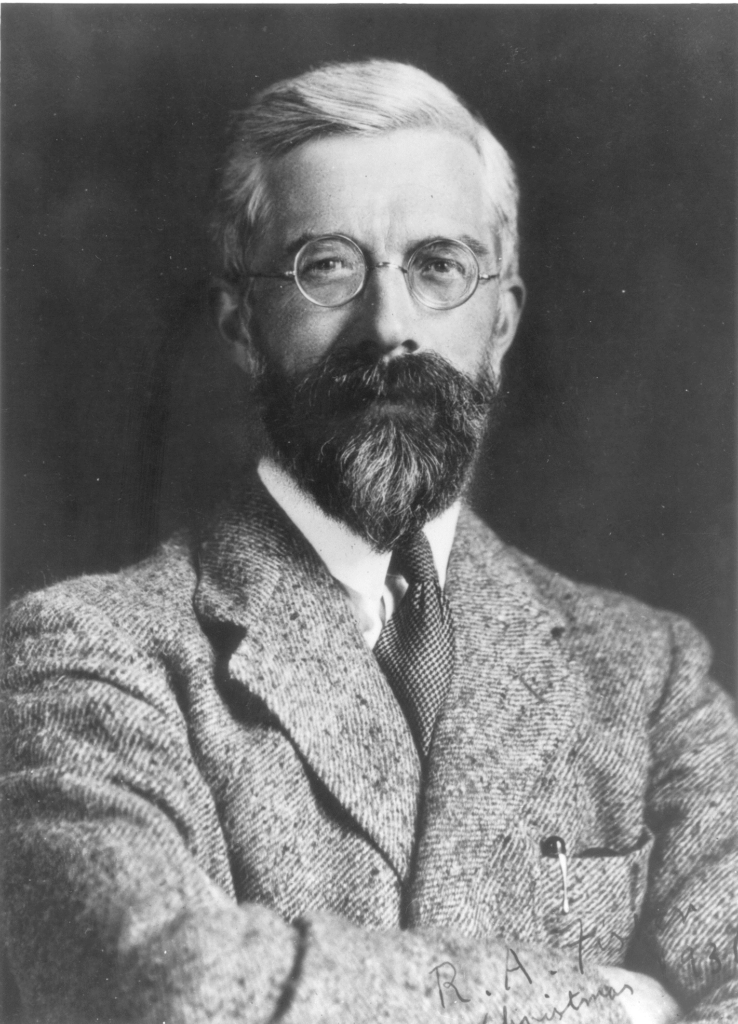
As a Ph.D. student and later an early career scientist, the story of Sir Ronald Fisher, population genetics, and eugenics was and is somewhat reassuring.
No matter how brilliant, well-connected, and critical to the development of science, superstars could be and often are fundamentally wrong in the most trivial fashion. Their prior achievements don’t give magical immunity to new errors, especially if those errors support their ego.
Such wrongness can and must be called out.
Or their scientific field will go from revered to scorned, and any advances – delayed by decades and decades.
Like it happened in theoretical population genetics starting from 1950s.
In ML and AI, this time to call out the wrongness of superstars is now.
Else this field won’t be blooming for long.