The “Fake News” as a term and a concept became ubiquitous ever since Trump used it in September 2016 to dismiss accusations of sexual assault against him.
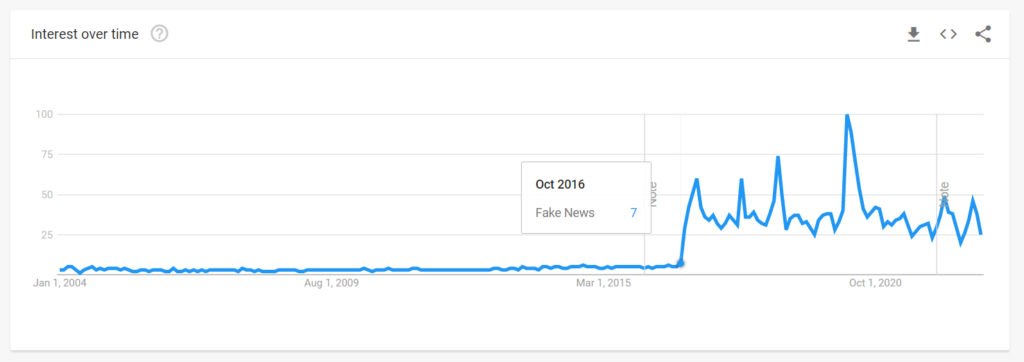
Unfortunately, that expression stuck ever since and is now used at large, including by experts in disinformation in their scientific literature.
This is highly problematic.
The ongoing common usage of “Fake News” term is harmful to healthy public discourse and research into misleading information.
Terminology forces a mindframe
Despite what his political opponents wanted to believe, Trump and his allies (notably Steve Bannon) are far from being dumb. While they are con men, they mastered the art of switching public attention away from topics that don’t suit them.
Using a specific vocabulary and terms that “stick” with the public is one of them, and the famous “covfefe” tweet is arguably the most egregious example of it.
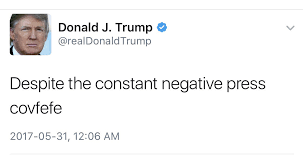
While many people still remember the term and the tweet itself, very few realized that it switched over the news cycle away from a bunch of seriously problematic stories for Trump, namely, Michael Flynn pleading the Fifth, Trump’s “deal” with Saudi Arabia, and massive cuts to science and education.
And the trick did its job incredibly effectively:
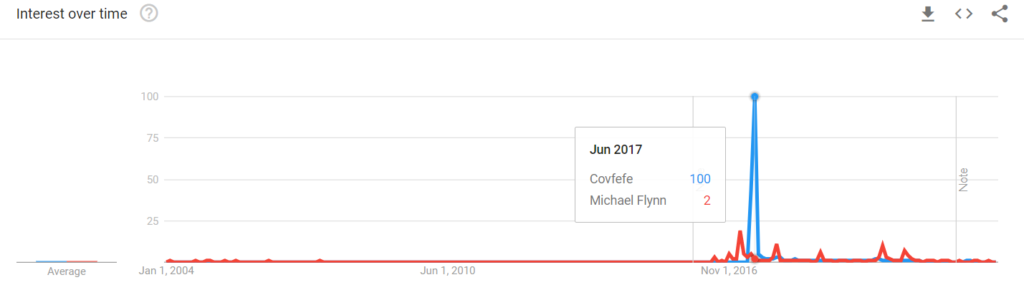
George Lakoff has warned us about conservatives using language to dominate politics and distort the perspective already in 2003.
“Covfefe” is a modern example of such usage. Michael Flynn pleading 5th and Saudi Arabia “deal” stories were pretty damaging to the image Trump has been trying to maintain with his followers. If gained enough momentum, they could have started eroding his base’s trust in him. A simple “spelling error from a president overtired from making all the hard decisions” can definitely be passed for “liberals nitpicking over nothing” – basically a mirror of Obama’s beige suit or latte salute.
“Fake News” is no different.
“Fake News” transforms public debate into a shouting match
A major issue with “Fake News” is that it stops a public debate and transforms it into a screaming match, based on loyalty. Trump says that accusations of rape against him and the “grab them by the pussy” tape are “Fake News”. Hilary says that his claims of being a billionaire and a great businessman are “Fake News”.
And that’s where the debate stops. Most people will just get offended that the other side is calling obviously true things “Fake News” or persisting in their belief that obviously “Fake News” are real. And their decision regarding what to go is already predetermined by prior beliefs, partisanship, and memes.
Which is exactly what you want when you know in advance that you are in the wrong and will be losing in a proper civil debate.
Which is Trump’s case. Hence the “Fake News”
And it is exactly the opposite of what you want when you are in the right and know you will win over people’s opinions if you have a chance to get their attention.
Which is the case for investigative journalists, scientists, civil rights activists, and saner politicians.
And yet, for whatever bloody reason the latter decided to get on with and keep using the “Fake News” term.
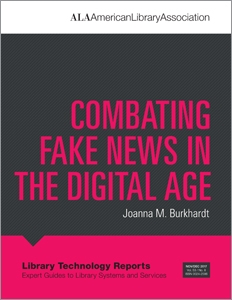
And by doing it, surrendered any edge their position might have had from being rooted in reality.
Just to appropriate a catchy term, even though it is meaningless and can be both a powerful statement and a powerless cry depending on how you see the person saying it.
Major journals publishing an in-depth investigation? Fake news by corrupt media!
Scientific journal publishing a long-awaited scientific article suggesting climate change is accelerating and is even worse than what we expected? Fake news by climate shills!
Are doctors warning about pandemics? Fake news by the deep state looking to undermine the amazing record of president Trump on job creation and economic growth!
Russian media outlets writing a story about the danger of western vaccines? Fake news! … Oh, wait, no, it’s just leftist cultural Marxists propaganda being countered by a neutral outlet!
Basically, by leaving no space for discussion, disagreement, nuance, or even a clear statement, “Fake News” is a rallying cry that precludes civil public debate and bars any chance to convince the other side.
“Fake News” is harmful to public debate.
“Fake News” is too nebulous for scientific research
Words have definitions. Definitions have meanings. Meanings have implications.
“Fake News” is a catchphrase and has no attached definitions. It is useless for scientific process.
How do you define “Fake” and how do you define “News”?
- Is an article written by a journalist lost in scientific jargon that has some things wrong “Fake News”?
- Is an information operation by a state-sponsored APT using puppet social media profiles to create a sentiment “Fake News”?
- Is yellow press with scandalously over-blown titles “Fake News”?
- How about a scientific article written with egregious errors that have results currently relevant to an ongoing pandemic – is it “Fake News” too?
- Are summaries and recommendations by generative language models such as GPT-Chat “Fake News”, even though it is trying its best to give an accurate answer, but is limited by its architecture?
None of those questions have an answer, or rather answer varies depending on the paper and the paper’s authors’ interests. “Fake News” lacks an agreed-upon operational definition and is too nebulous for any useful usage.
“Fake News” is harmful to the research on public discourse and unauthentic information spread.
Ironic usage is not a valid excuse
An excuse for keeping using the “Fake News” around is that it’s ironic, used in the second degree, humor, riding on the virality wave or calling the calling the king naked.
Not to sound like fun police, but none of them are valid excuses. While the initial use might not be in the first degree, its use still affects thinking patterns and will stick. Eventually, the impact of usage of the term will become more and more natural and start affecting thought patterns, until in a moment of inattention it is used in first-degree.
Eventually “Fake News” becomes a first-degree familiar concept and starts being consistently used.
The harm of “Fake News” occurs regardless of initial reasons to use it.
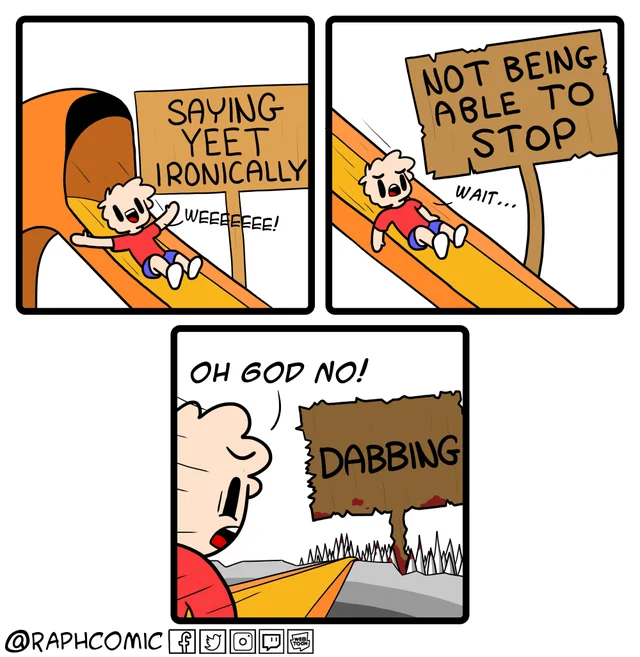
A better language to talk about misleading information
To add insult to injury, before “Fake News” took over we already had a good vocabulary to talk about misleading information.
Specifically, misinformation and disinformation.
Intention.
Not all misleading information is generated intentionally. In some cases a topic is complex can just be plain hard, and the details making a conclusion valid or invalid – a subject of ongoing debate. Is wine good or bad for health? How about coffee? Cheese? Tea? With about 50 000 headlines claiming both, including within scientific, peer-reviewed publications, it makes sense that a blogger without a scientific background gets confused and ends up writing a misleading summary of their research.
That’s misinformation.
While it can be pretty damaging and lethal (cf alt-med narrative amplification), it is not intentional, meaning the proper response to it is education and clarification.
Conversely, disinformation is intentional, with those generating and spreading it knowing better, but seeking to mislead. No amount of education and clarification will change their narrative, just wear out those trying to elucidate things.
Means
Logical Fallacies are used and called out on the internet to the point of becoming a meme and a “logical fallacy logical fallacy” becoming a thing.
While they are easy to understand and detect, the reality is that there are way more means to err and mislead. Several excellent books have been written on the topic, with my favorites being Carl Bergstrom’s “Calling Bullshit”, Dave Levitan’s “Not a Scientist” and Cathy O’Neil’s “Weapons of Math Destruction“.
However, one of the critical differences between just calling out misinformation/disinformation from “Fake News” is explaining the presumed mechanism. It can be elaborate as “exploited Simpson’s paradox in clinical trial exclusion criteria” or as simple as “lie by omission” or “falsified data by image manipulation“.
But it has to be there to engage in a discussion or at least to get the questioning and thinking process going.
Vector.
No matter the intention or the mechanism of delivery, some of the counterfactual information has a stronger impact than others. A yellow press news article, minor influencer TikTok, YouTube, or a Twitter post are different in their nature but are about as likely to be labeled as untrustworthy. Reputable specialized media news articles or scientific journal publications are on the opposite side of the spectrum of trustworthiness, even though they need amplification to reach a sufficient audience to have an impact. Finally, major cable news segments, or a declarations by a notable public official – eg a head of state – are a whole other thing, percieved as both trustwrothy and reaching a large audience. Calling it out and countering it would require an uphill battle against authority and reputation and a much more detailed and in-depth explanation of misleading aspects.
More importantly, it also provides a context for those trying to spread misleading information. Claiming the world is run by reptilians because a TikToker posted a video about it is on the mostly risible side. Claiming that a public official committed fraud because a major newspaper published an investigative piece is on a much less risible one.
Motivation / Causes.
Finally, a critical part of either defeating disinformation or addressing misinformation is to understand the motivation behind the creators of the former and the causes of error of the latter.
This is a bit harder when it comes to the civil discourse, given that accusations are easy to make and hard to accept. It is however critical to enable research and investigation, although subject to political considerations – in the same way as attribution is in infosec.
Disinformation is pretty clear-cut. Gains can be financial, political, reputational, or military gain, but it’s more of the exact mechanism envisioned by the malicious actor that’s harder to identify and address. They are still important to understand to effectively counter it.
Misinformation is less clear-cut. Since there is no intention and errors are organic, the reasons they emerged are likely to be more convoluted. It can be overlooking primary sources, wrong statistical tests, forgetting about survivorship bias or other implicit selection present in the sample, or lack of expertise to properly evaluate the primary sources. Or likely all of the above combined. They are still important to understand how misinformation emerges and spreads and to stop it.
A couple of examples of better language.
HCQ for COVID from Didier Raoult’s lab
This is a disinformation scientific article, where misleading conclusions were generated by manipulating the exclusion criteria in medical trials and a phony review, motivated by a reputational, political, and likely financial gain.
Chuck Yager’s support of presidential candidate Trump
This is a disinformation social media post, that operated by impersonation of a public figure, motivated by adding credibility to Trump as presidential candidate for political gain.
DeepFake of Zelensky giving the order to surrender
This is a disinformation video on a national news channel, that was generated using deep generative learning (DeepFake) and injected using a cyber-attack, motivated by immediate military gain resulting from UA soldiers surrendering or at least leaving their positions.
Vaccine proponents are pharma shills
This is a piece of disinformation news, blog posts, and scientific articles, that were generated by manipulating data and hiding the truth, motivated by financial gains from money given by pharma.
Vaccine opponents make money from the controversy
This is disinformation blog posts, news articles, and videos, that were generated by selecting results and fabricating data, motivated by financial gains from sold books, films, convention tickets, and alt-medical remedies.
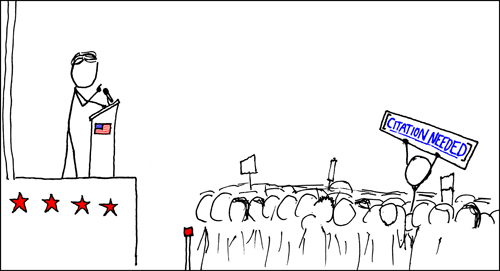
Better language leads to a better discussion
The five examples above got you screaming for references for each part of the statement, whether you disagree with them or agree with them.
Good.
That’s the whole point.
Same statements, but as “<X> IS FAKE NEWS” would have led to no additional discussion and just an agreement/disagreement and a slight annoyance.
A single, four-part statement regarding presumably misleading information is harder to form, especially if references are included but is also hard to deny, refute, and just upon being stated will open a door to hesitation and investigation.
Which is the opposite of “Fake News”. Which is what we want.
“Fake News” should be considered harmful and its use – abandoned.
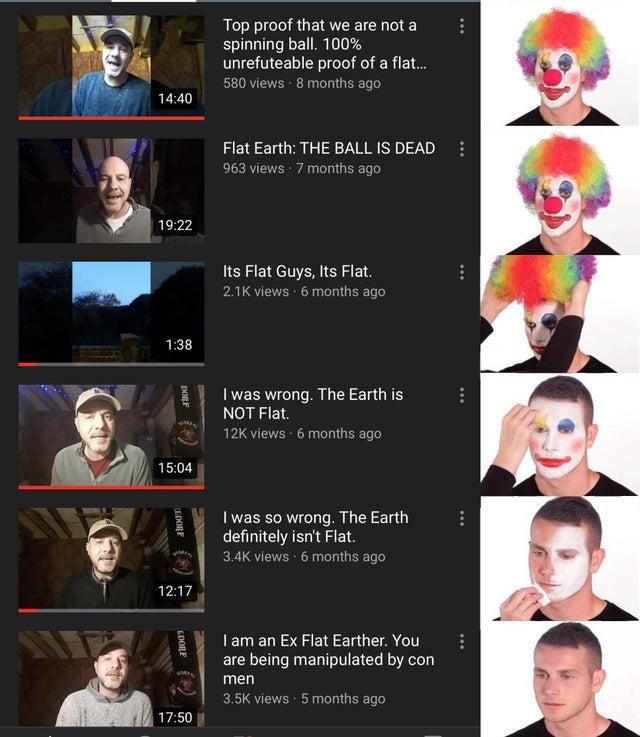